The healthcare industry is increasingly relying on technology to manage, store and share sensitive patient data. While the use of health tech has made healthcare more efficient, it has also created new security challenges. The security of sensitive patient data is a top concern for healthcare providers, policymakers, and patients. In this essay, we will explore how health tech can ensure the data in use is securely protected.
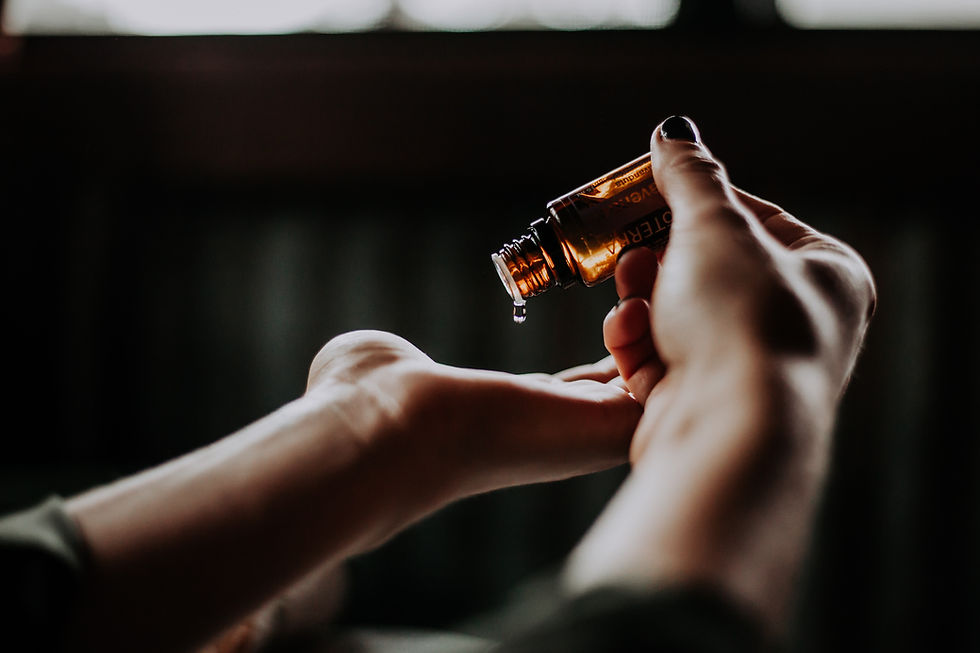
The Importance of Data Security in Healthcare
Data security is crucial in healthcare as it ensures that patient information is kept confidential and protected from unauthorized access. Healthcare data is highly sensitive, and any breach of privacy can have severe consequences for patients. It can lead to identity theft, medical fraud, and even harm to patients if their medical records are altered or deleted. This is why healthcare organizations need to take data security seriously and implement robust security measures to protect patient data.
Health Tech Solutions for Data Security
Health tech can play a significant role in ensuring that patient data is securely protected. One approach to secure healthcare data is to use encryption methods. Encryption makes data unreadable to unauthorized users, making it more difficult for them to access sensitive patient information. It involves converting plain text into a coded message that only authorized users can understand. Encryption protocols are simple to implement and are widely available. This ensures that even if hackers get access to the data, they cannot read it.
Another approach is to use two-factor authentication, which requires users to provide two forms of identification before accessing patient data. This adds an extra layer of security and makes it more challenging for unauthorized users to access patient data. For instance, users may be required to provide a password and a fingerprint scan before accessing patient data.
Another approach to secure healthcare data is to implement robust access controls. Access controls can limit the number of people who have access to sensitive patient data. Access controls can also restrict the type of data that users can access, ensuring that only authorized users can view and modify sensitive patient data. For instance, healthcare organizations can implement role-based access controls, where users are only given access to the data that is necessary for their job.
The Challenge of Data Sharing
While health tech can ensure that patient data is securely protected within a healthcare organization, data sharing between healthcare providers and other third-party organizations can present new security challenges. To address this challenge, healthcare providers are implementing secure data-sharing protocols that ensure that patient data in transit is only shared with authorized users. These protocols can include secure file transfers, secure messaging, and secure APIs. Implementation of the above-mentioned methods leads to the technological solution to the given issue. Health tech can play a significant role in ensuring the data in transit is securely protected. However, it is essential to implement the right security measures to protect sensitive patient data from unauthorized access. The healthcare industry must continue to invest in secure data-sharing protocols, encryption technology, two-factor authentication, and access controls to ensure that patient data is kept confidential and protected from cyber threats. By doing so, health tech can continue to improve healthcare while protecting the privacy and security of patients. It is also important to educate healthcare providers and patients about the importance of data security and the potential risks of data breaches. By raising awareness and implementing the right security measures, the healthcare industry can ensure that patient data is kept confidential and protected from unauthorized access.
The Role of Artificial Intelligence and Machine Learning in Data Security
Numerous AI startups and researchers at the world's leading universities are seeing significant advancements in early disease detection using AI and ML. In order for such research to be effective, access to patients' data is crucial.
AI and ML have great potential as early disease detection and predictive models. With the increasing amount of health data being collected and advancements in AI and ML, these technologies have become valuable tools for healthcare professionals and researchers. Early disease detection is crucial for the effective treatment and prevention of diseases. AI and ML algorithms can analyze large amounts of patient data, such as medical history, lab results, and imaging scans, to identify patterns and detect early signs of disease. These algorithms can also be trained to recognize subtle changes that may indicate disease progression or response to treatment. Predictive models based on AI and ML can also be used to identify individuals who are at high risk of developing certain diseases. By analyzing multiple factors such as age, genetics, lifestyle, and environmental factors, these models can identify individuals who are most likely to develop a particular disease. This allows healthcare professionals to intervene early and provide personalized preventive care.
Some examples of AI and ML in early disease detection and predictive models include:
Using AI algorithms to analyze medical imaging scans, such as mammograms, to detect breast cancer at an early stage.
Developing predictive models based on genetic and lifestyle factors to identify individuals at high risk of developing type 2 diabetes.
Using AI-powered wearables to monitor changes in heart rate, blood pressure, and other vital signs, to predict and prevent heart disease.
Developing AI algorithms to analyze electronic health records (EHRs) and predict patient outcomes, such as readmission rates and mortality rates.
While AI and ML have the potential to revolutionize early disease detection and predictive models, there are still some challenges that need to be addressed, such as privacy concerns and ensuring the accuracy and reliability of algorithms. However, with continued advancements and research, AI and ML are likely to become even more valuable tools in healthcare.
AI and ML have enormous potential to improve data security in healthcare. These technologies can help healthcare organizations identify and respond to potential security threats in real-time. For instance, AI and ML can be used to analyze user behavior and detect suspicious activity such as unauthorized access attempts, abnormal data transfers, or unusual data access patterns. This can help healthcare organizations take proactive measures to prevent data breaches and ensure that sensitive patient data is protected. For instance, hospitals are full of patient data that has to be protected due to their highly sensitive nature of it.
AI and ML can also be used to automate security management tasks, such as monitoring access logs, tracking changes in user permissions, and enforcing data retention policies. This can help healthcare organizations reduce the risk of human error, ensure that security policies are consistently enforced across the organization, and rise general safety.
An example would be a university research group working on early disease detection AI, that needs to access patients' data from hospitals to train and test their models. Using tech, one can deploy a trusted execution environment on the cloud, which enables such collaboration while ensuring full privacy of the data. One can also deploy the TEEs (trusted execution environment) within the hospital's network to ensure the data never leaves the premises.
Deploying a TEE is a lengthy and complicated process. The way you are protecting your data during processing is by isolating a part of the CPU where that processing is taking place, this is exactly what a TEE (often called an enclave) does. The problem is, that in order to provide such a level of data security, even the OS (operating system) cannot access the TEE. This means, that a TEE is not able to understand the code your application is written in. Several startups are tackling this problem by developing open-source libraries that help you deploy applications inside TEEs, but there is still progress to be made.
Using the mentioned technologies, data security problems at rest and in transit are already solved in the problem. However, in order for humanity to receive significant value from the application of AI and ML in healthcare, it is necessary to effectively protect data in use. The only viable method of such protection now is the use of Trusted execution environments based on confidential computing technology. The most famous example is Intel SGX. The technology is now very new and difficult to use, but it is developing very rapidly.
Future Directions for Health Tech and Data Security
As health tech continues to advance, healthcare organizations must continue to adapt and evolve their security measures to keep up with the changing threat landscape. One area of innovation is the use of confidential computing. Confidential computing refers to the ability to process data in an encrypted form, thus preventing unauthorized access to the data. This technology ensures that data is protected even when it is being processed. Confidential computing is achieved by using hardware security solutions that allow data to be processed in a trusted environment. The hardware is designed to protect data from software attacks, including those that exploit vulnerabilities in the operating system. Confidential computing offers numerous benefits, including enhanced data privacy and security. With confidential computing, sensitive data is protected from unauthorized access, reducing the risk of data breaches. Confidential computing also enables greater control over data, allowing users to determine who has access to their data and how it is processed. Additionally, confidential computing can facilitate secure data sharing between organizations, enabling collaboration without compromising data privacy.
Another area of innovation is the development of secure cloud-based platforms for storing and sharing patient data. Cloud-based platforms can provide secure and scalable solutions for healthcare organizations. With its work, Decision 3 enables to storage and share large amounts of patient data while maintaining high levels of security.
Conclusion
In conclusion, health tech has the potential to improve healthcare by making it more efficient and accessible. However, the security of sensitive patient data is a top concern for healthcare providers, policymakers, and patients. Health tech solutions such as encryption technology, two-factor authentication, access controls, AI, and ML can help ensure that patient data is securely protected. As health tech continues to advance, healthcare organizations must continue to adapt and evolve their security measures to keep up with the changing threat landscape. By investing in secure data-sharing protocols, cloud-based platforms, and especially confidential computing, healthcare organizations can ensure that patient data is kept confidential and protected from cyber threats.